Reinventing telecoms with generative AI
CSPs at a crossroads
Like most business sectors, the telecommunications industry has eagerly pursued the groundbreaking potential of generative AI to reimagine its future. In a recent TM Forum report, communications service providers (CSPs) identified more than 100 individual use cases for using the technology. A new IBM Institute for Business Value (IBM IBV) survey of 300 global telecom leaders shows the same enthusiasm—but tempered with some concerns.
First, the positives. CSPs are committing resources: gen AI spend as a percentage of total AI spend is expected to grow 37% over the next two years. And they are assessing and deploying use cases across key business areas.
CSPs are assessing and deploying gen AI use cases across multiple business areas.
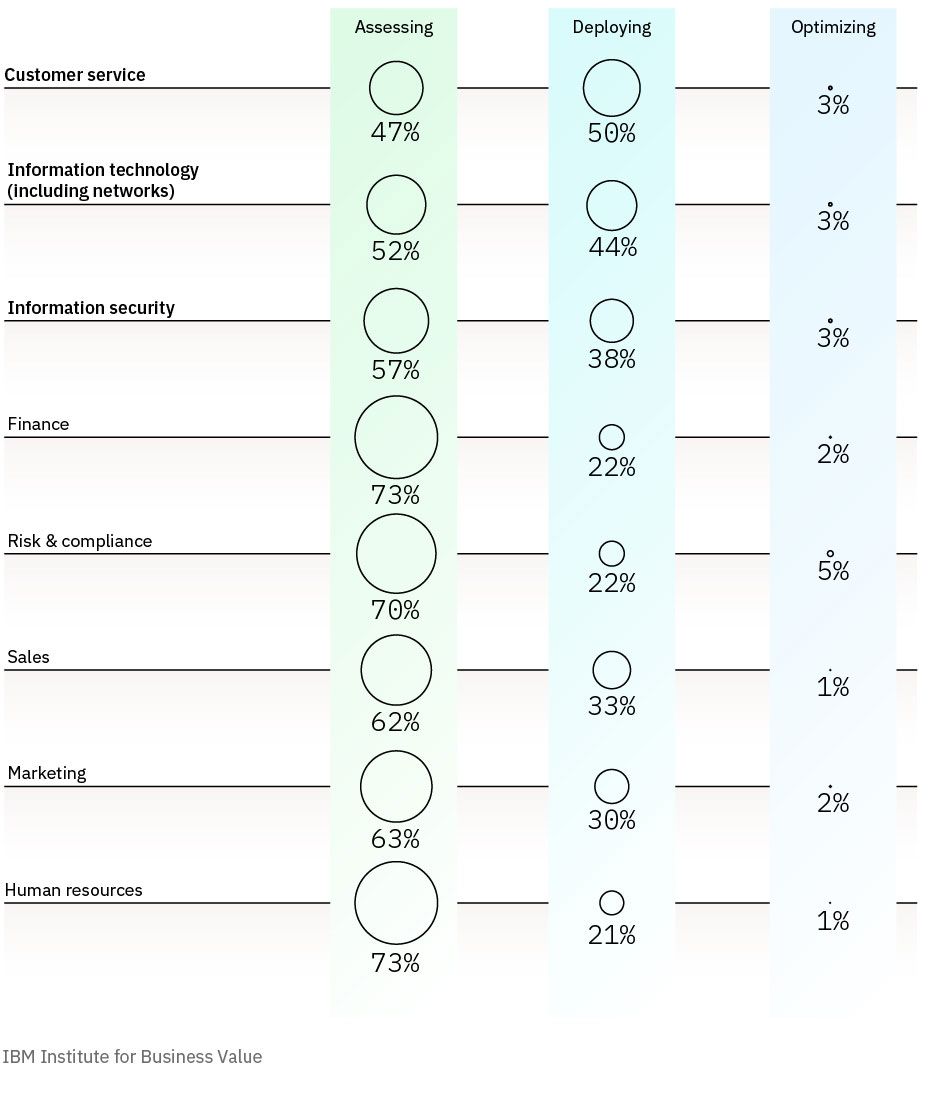
Telecom executives also have high expectations for the potential business impact of these use cases. At closer inspection, though, gen AI is posing a conundrum for industry leaders. Less than one-fourth of our survey respondents say they have defined how the technology can differentiate their enterprise strategy.
Telecom executives anticipate generative AI will create new opportunities within and outside the industry over the next three years.
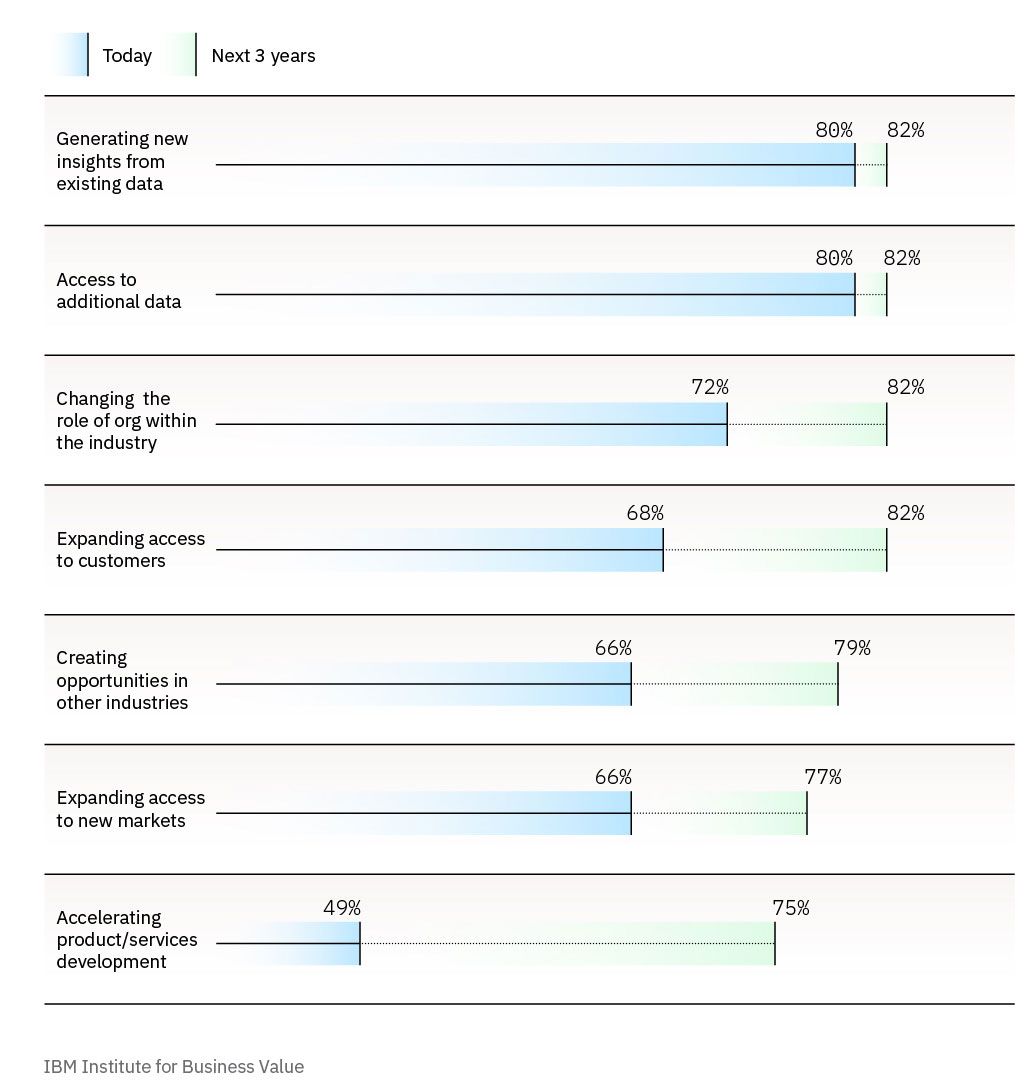
“The pace of change has been so rapid, there has not been enough time to be strategic, although it is necessary to be strategic” observes Ken Komazawa, Vice President, IT Strategy Office, NTT.
In fact, most executives don’t see their organizations as fully ready to adopt gen AI: 45% grade their readiness as fair with only one-third grading it as good. They cite resource constraints—both talent and technical—among their top adoption barriers.
Executives are wrestling with the value and viability of generative AI.
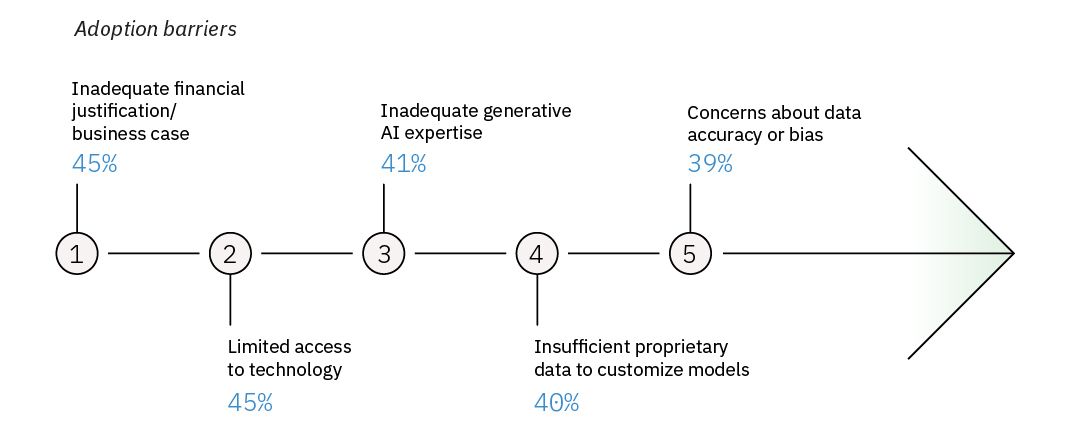
Additionally, more than half (56%) of those surveyed say they don’t have a mature, defined approach for adopting the technology. Bharti Airtel CIO Pradipt Kapoor is concerned CSPs are missing the breadth and significance of the many choices they need to make. “Large enterprises don’t understand the consequences of the decisions that are being made,” he says. “Where to invest? What infrastructure to procure? Which model to use? What’s the cost-to-value equation?”
To address this intense uncertainty, the IBM IBV partnered with TM Forum to identify seven key aspects of generative AI adoption that telecom executives should consider. Understanding and addressing these factors can help organizations move quickly—and judiciously—from experimentation to productive, value-creating generative AI initiatives.
“Speed without direction is short-sighted,” says e&’s Group Chief AI and Data Officer, Dena Almansoori. “The true potential of AI lies in a thoughtful integration into the fabric of the business, ensuring that it supports our long-term goals and strengthens our position over the long run. The opportunity is not AI itself but what we ultimately build with it.”
That means taking an AI-first approach to strategically transform the business. The following key considerations can help CSPs shift from cautious to confident in their enterprise adoption and deployment of generative AI.
7 keys to generative AI adoption
![]() | 1. Choose the right gen AI use cases. |
CSPs are exploring a vast array of gen AI use cases, with leading CSPs strategically identifying quick wins and longer-term opportunities. But how do organizations choose what makes the most sense to focus on?
One approach: begin with low-risk experiments that increase employee efficiency, such as using gen AI to create content for marketing campaigns or summarize documents. But the real challenge comes in identifying—and deploying—those select use cases that can transform operations and customer experiences.
For our respondents, decisions start with key criteria for success. Given the resource concerns cited as adoption barriers, it is not surprising to see maturity of ecosystem partners, implementation complexity, and availability of skills among the top hurdles. Respondents also are focused on finding those use cases that can deliver the most value based on strategic alignment, financial ROI, and benefits to end users.
Executives evaluate gen AI use cases based on six key criteria.
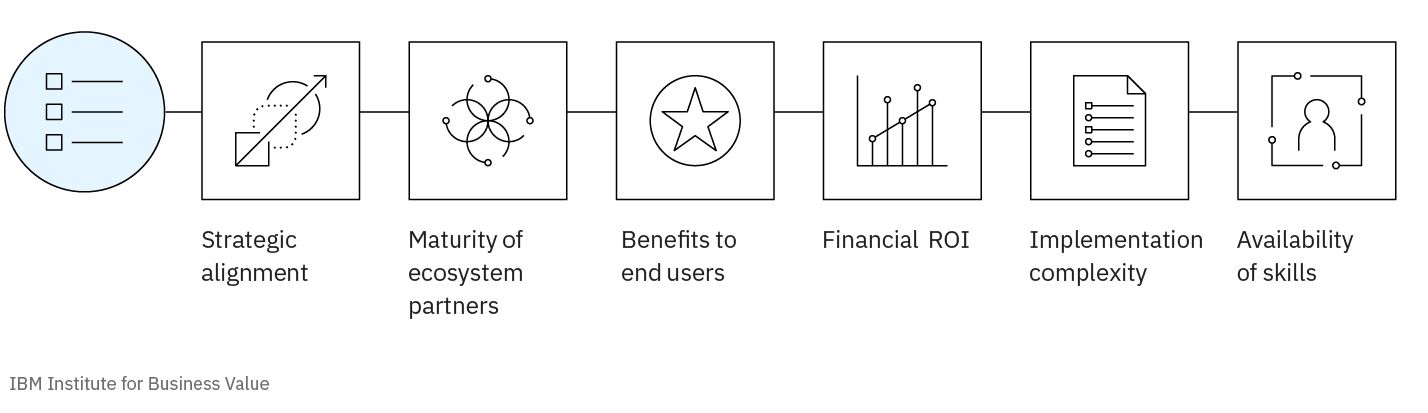
As shown earlier, customer service, IT (including network operations), and information security have emerged as the leading business areas where gen AI is being deployed. Within these, respondents are evaluating use cases based on value and feasibility. For example, in customer service, configuring chatbots and augmenting knowledge search rank the highest.
CSPs are identifying the most promising customer service use cases based on value and feasibility.
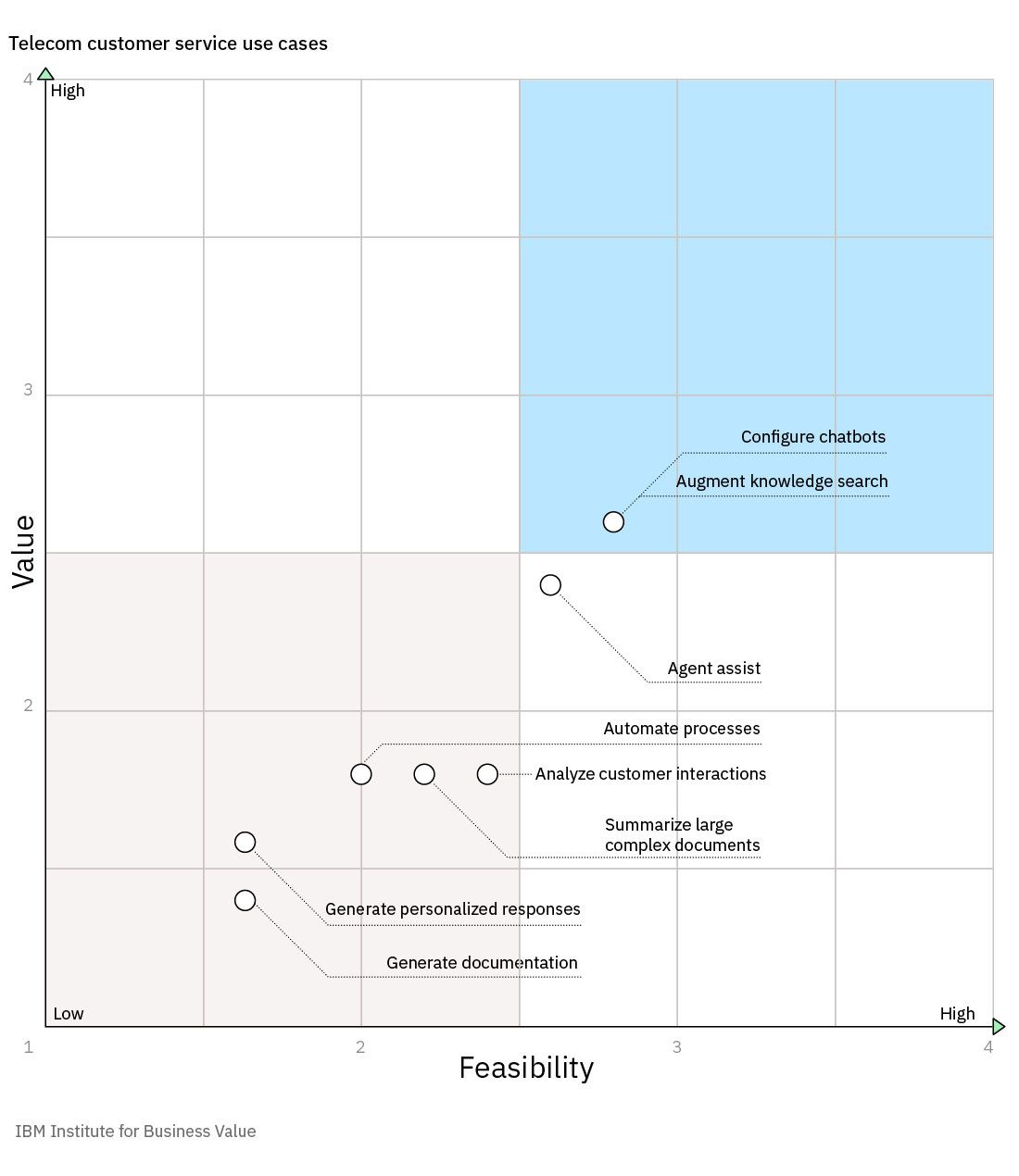
When considering which use cases to pursue, organizations should identify those generative AI capabilities that can be leveraged within multiple areas of the business. Take augmenting knowledge searches with gen AI as an example. Our analysis shows it as a strong use case in customer service, IT/network operations, and information security, but it could also pertain to human resources or procurement or sales. This use case applies gen AI algorithms to improve the search functionality of existing knowledge bases. Because the algorithm continuously learns from its interactions, results become more personalized and enable the user to be more proactive. When integrated into an organization’s platforms, augmented knowledge searches help users find more accurate information quicker.
As use cases are vetted, NTT’s Komazawa advises that a cross-functional team is vital. “In the business sector, sales and engineering need to be involved. In management, you need governance, legal, and intellectual property,” he says.
The results of our analysis may not apply to every organization, of course. For instance, Bharti Airtel’s Kapoor cautions that CSPs should not assume customer service is the best starting point. As expensive as generative AI can be, it isn’t always the correct choice for cost-cutting goals, especially when considering a company’s size and region.
Kapoor suggests that long-term value can often be found in better mapping of customer and network data, and then building enterprise generative AI use cases on top of that. “It goes back to data monetization, which telcos have struggled with,” he explains. “They have been sitting on a lot of data, but the aggregators have traditionally made a lot more money from it. Telcos haven’t cracked the code for how to share data with each other without an aggregator in play.”
Questions to ask:
|
Perspective
While some operators centralize their AI efforts, Bharti Artel CIO Pradipt Kapoor has taken a successful federated approach. “At Airtel, we believe in the transformative power of AI and gen AI,” he says. “Like with any new technology, there is a cycle of awareness, evangelizing, and training that must be followed.”
He notes that they educated every department in Airtel—supply chain, finance, marketing, HR, and more—on the technology, presenting the concepts as well as the art of the possible through demos and hands-on experiences. “We have also tried to templatize the process for idea generation with the right kinds of questions to identify business value use cases,” he explains. “Then there was a partnership with our digital (product and engineering) teams to see what they thought they could do given the data and technology we have.”
With a focus on value creation in either customer service, cost and efficiency improvements, or driving growth, the company now has 28 to 35 gen AI use cases either in or near production. These include:
- An employee chatbot allows personnel to ask questions such as “how much leave do I have” or “what does the parental policy mean?”
- Call transcripts and summaries are auto generated for all incoming contact center calls and automatically uploaded to the customer interaction history. Taking it a step further, sentiment analysis is also being run on the calls. These initiatives have significantly improved average handling time and call quality.
- Marketing is using a generic LLM to generate hyper-localized content and offers.
- Outgoing collection calls for some bills are now gen AI-based voice bot calls. This effort is showing early success, bumping the outstanding bill payment rate from 60% for a team of 100 to 64% for the voice bot.
- Developers are using gen AI to help generate test cases and code.
Source: Interview with Bharti Airtel CIO, Pradipt Kapoor. April 19, 2024.
![]() | 2. Identify and assess data. |
Trusted, high-quality data is the lifeblood of generative AI. That requires CSPs to consider both the data they need and the infrastructure supporting it. “Telcos possess vast amounts of structured and unstructured data, ideal for successfully harnessing third-party gen AI models,” says e&’s Almansoori. “However, this data advantage can only be truly leveraged if they have the right tools and talent in place.”
CSPs should first look at whether they have accurate, complete, and current data that is relevant to each use case. This may involve collecting and integrating data from various sources, such as customer interactions, network performance metrics, and external data providers. It could also involve non-operator data. For example, weather and traffic data can be used to predict network issues, or social media data about competitor’s outages can feed marketing campaigns.
As they evaluate this data, CSPs must consider data privacy and security regulations, which affect decisions on how customer data is used, secured, and stored (such as on premises or in the cloud). In fact, 71% of executives are concerned about data laws that restrict how they move or use data in their gen AI models. And with more than one-third of respondents (37%) wary about cybersecurity hackers manipulating their gen AI models, they also need to focus on fundamental data security principles to protect both the inputs and outputs of the models.
CSPs must guard against potential biases in the data as well. That means tracking the lineage of the data used in the models and ensuring it represents a range of customers, networks, and scenarios.
Finally, a CSP’s data infrastructure is critical to supporting the integration of data from disparate sources. Unfortunately, that is a weakness for many CSPs. Bharti Airtel’s Kapoor observes, “I come from the school of thought that most telcos think of data infrastructure as a data lake for reporting,” he says. “But I don’t think too many telcos are thinking about going beyond a data lake to a data platform that can power business and use cases.”
Our data suggests the same: only 30% of respondents expect generative AI will significantly accelerate their engagement with data and analytics platforms over the next three years. And 60% of executives say their data integration capabilities are immature or basic.
These limitations may make it difficult for CSPs to pull in the data that best aligns with their gen AI use cases. A solution such as a data fabric architecture can support the needed end-to-end integration of multiple data pipelines and cloud environments.
“Good-quality data clearly catalogued and understood is the fuel for gen AI and AI,” says Kapoor. “People forget that. Invest in your data platform first.”
Questions to ask:
|
![]() | 3. Choose the generative AI model(s) and understand model training requirements. |
Generative AI models are evolving rapidly, offering many options to CSPs. While last year, attention focused on multipurpose large language models (LLMs), this year has seen the growth of small language models (SLMs), which can be much more cost-effective and relevant. Organizations can start with an LLM for experimentation and then move to an SLM as they refine their use cases.
CSPs can also choose from proprietary models, such as OpenAI’s ChatGPT and Anthropic’s Claude, and open models, such as Meta’s Llama and Mistral AI’s Mixtral. Some models are multimodal, designed to process and generate data in text, image, and even audio formats. Industry-specific foundation models are also under development.
NTT’s Komazawa says it’s important for the models to meet the unique needs of the Japanese market, including their organization, and they have, in fact, built their own LLM. “Geopolitically, Japan is a unique environment with its own language and culture,” he explains. But he also expects they will use multiple LLMs.
Choosing one or more models is not straightforward. Ultimately, CSPs will likely need multiple models—offering greater accuracy and multiple capabilities. But they must be aware of the increased costs and complexity and guard against accruing technical debt through the uncontrolled purchase and deployment of different AI models and services across the organization.
At the same time, they should avoid getting locked in with a single vendor, particularly given the pace of change. They could find themselves unable to switch easily to a service provider that offers the latest technology advancements.
As CSPs evaluate their model choices, they also must consider how the model will need to be adapted to meet their needs more precisely. The most widely used techniques include:
- Prompt engineering. This process involves designing and testing different prompts or inputs to see how a model performs on a specific task with the goal of finding the prompt that results in a high-quality response. Prompt engineering is a cost-effective way to improve a model’s performance on a specific task, but outputs are limited to what the model has learned in its initial training. The technique doesn’t add more specific or new information to the model.
- RAG (Retrieval Augmented Generation). This technique leverages external data sources to provide more up-to-date, relevant information but is more costly than prompt engineering. It does require integration between the model and the system holding the additional data.
- Fine-tuning. This method adapts a pre-trained language model by training it on a small amount of task-specific data, resulting in more accurate outputs. This process can be effective for a wide range of natural language processing tasks, such as text classification, sentiment analysis, question answering, and more. Fine-tuning requires significant compute power and time, as well as technical skills and a curated data set, making it more expensive but less pricey than building custom models.
Many CSPs operate in environments that mix old and new technologies, which can present integration challenges for gen AI models. “While the benefits of AI are clear, its adoption requires properly handling interoperability with legacy systems upfront,” notes e&’s Almansoori. “Strategic planning around needs, streamlining legacy infrastructure, and a practical phased approach to integration are key.”
Questions to ask:
|
Perspective
The rapid evolution of AI is transforming the approach to complex problems in the telecom industry. IBM Research® is pioneering the application of foundation models on network data, a cutting-edge AI approach that leverages extensive, unlabeled data sets to drive innovation.
In collaboration with a leading Tier-1 CSP, the team achieved a breakthrough in optimizing radio access network (RAN) parameters in metro settings. By tailoring and fine-tuning a foundation model to work with data from mobile networks, they’ve demonstrated significant improvements in performance and coverage. The proof of concept showed that this AI-powered approach can accurately predict downlink throughput, enhance service quality, and boost network performance scores (NPS). Notably, the model outperformed the efficiency of traditional machine learning approaches such as XGBoost.
The trained foundation model can be deployed in multiple use cases, including:
- Bad cell identifier. Identifying underperforming cells to optimize network resources.
- Optimal configuration parameters. Determining the ideal configuration settings for maximum network efficiency.
- Multivariate analysis. Evaluating the impact of new feature deployments on network performance.
- Network simulator. Using the foundation model to predict the effects of configuration changes, enabling iterative tuning of parameters to optimize network KPIs. This simulation-driven approach has the potential to revolutionize the way CSPs optimize network performance, enabling telecom operators to make data-driven decisions and stay ahead of the competition.
Source: Interview with IBM Research, April 2024.
![]() | 4. Develop a business case. |
Generative AI solutions are complex and expensive—not a simple add-on to an existing system. The technology requires CSPs to consider the costs across the full solution lifecycle. These include:
- Inference costs. These reflect the expense of the model to generate a response to a prompt. This process requires significant compute resources.
- Fine-tuning costs. These will vary based on the size and complexity of the model, the amount of data being used, and the number of iterations required for training.
- Cloud expenses. These extend beyond the cost to host gen AI applications. Organizations must factor in the additional storage costs for the data being generated by the applications. Cloud and legacy infrastructure strategies must also be revisited to make sure they are cost-effective for generative AI solutions.
- Deployment costs. These include the expenses from deploying solutions in multiple regions, such as server hosting.
- Talent costs. Short- and long-term plans for talent must be considered, including reskilling and hiring priorities for developers, data managers, and security teams; beefing up security education so that employees are aware of the increased risks from generative AI; and determining the role of human feedback in model training.
The second half of the cost-benefit equation can be tougher to calculate. For the telecom industry, generative AI can fuel business value through productivity improvements, improved risk management, and compelling products/services. For example, IBM pilots of generative AI solutions have shown the cost of marketing content creation can be reduced up to 40%, potential cyber threats can be contained up to eight times faster, and automated customer service solutions deliver answers with up to 95% accuracy.1
To better understand the financial value of a solution, CSPs can use a value tree to assess how the solution can help grow revenue, manage costs, and mitigate risks. More specifically, they need to evaluate specific metrics such as percentage of new customers, percentage of revenue from new products, sales cycle times, returns on marketing investments, and average time to detect and respond to a cybersecurity incident, to name a few.
A value tree aligned to key business performance measures helps identify the financial value of a gen AI solution.
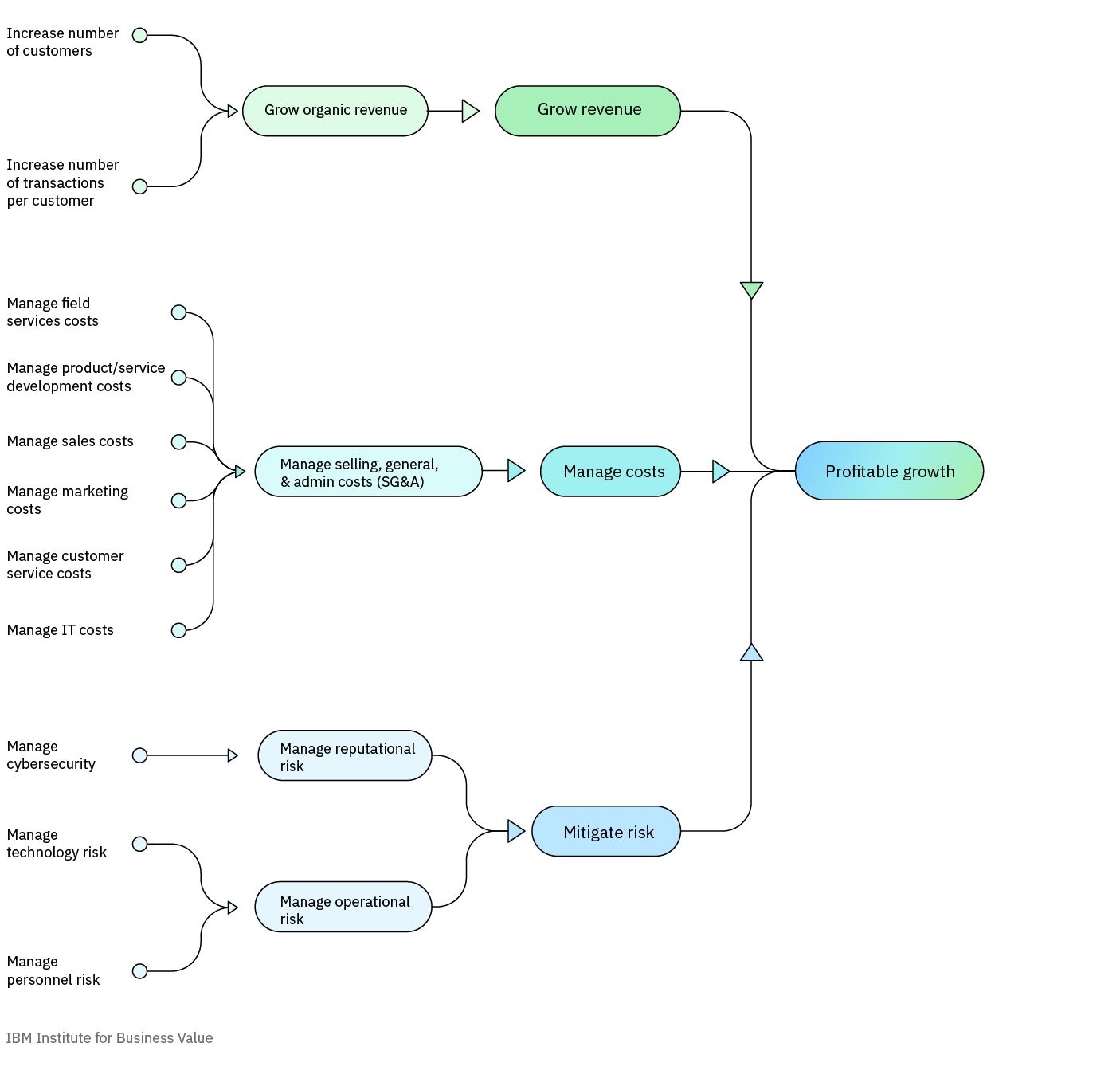
Source: IBM IBV Benchmark Program
Working through the value tree to build an effective business case requires strong relationships between technology and finance teams. “Close collaboration between technology leaders and finance teams allows for the translation of technical visions and metrics into clear business benefits and ROI projections while also optimizing investments and ensuring initiatives are structured properly to maximize ROI,” says e&’s Almansoori.
Questions to ask:
|
![]() | 5. Update AI governance. |
For AI initiatives to be successful, they must be trusted. AI governance reflects the guardrails that help ensure AI tools are behaving safely and ethically. Governance addresses the risks associated with AI, such as bias, discrimination, and harm to individuals, as well as being able to explain the outcomes.
“Data accuracy and bias concerns cannot be understated. We’ve seen increased focus from regulators and customers on issues like data sovereignty, privacy, and model explainability, given telecoms' unique access to personal digital information flows and network oversight role,” says e&’s Almansoori.
NTT’s Komazawa echoes the mandate for governance. “In the past, there were technologies expected to bring disruptive innovation, but we could not to come to the decision to deploy due to the governance issue,” he says.
Executives are confident in their traditional AI governance, with 78% saying they have established clear organizational structures, policies, and processes. This can serve as a solid foundation, but gen AI introduces new considerations. For example, to protect intellectual property, organizations need guardrails for how gen AI is used by employees, and governance should address the issues that can arise from using models pre-trained on large amounts of data that an organization has little control over.
As CSPs move forward with generative AI use cases, e&’s Almansoori suggests updating established traditional AI governance frameworks to address:
- An increased focus on explainability and transparency. Gen AI requires a deeper understanding of how the model arrives at the content it creates—text, code, or other media. CSPs need to be able to demonstrate the reasoning behind gen AI’s creations and mitigate biases in the training data.
- Unique risks associated with gen AI. Regulatory frameworks and standards are evolving to anticipate the risks from deepfakes and other forms of synthetic media used for malicious purposes. Limitations on specific gen AI functionalities and stricter oversight mechanisms may be on the table, and CSPs will need to comply.
- AI-human collaboration. As the nature of AI-human collaboration evolves, CSPs need guidelines to govern how humans and AI interact, how responsibility for decisions is attributed when AI assists, and how human oversight is retained in critical tasks.
- Data privacy and integrity. Generative AI’s dependency on high volumes of training data raises concerns about biases as well as unintentional use of copyrighted data. As data and models are chosen for use cases, CSPs need to assess the risks and understand their responsibilities for data privacy.
Prioritizing responsible AI principles and regulations is not just the right thing to do; it can give CSPs an advantage in the market. “When telco players make transparency, explainability, safety, and fairness core priorities from the start, they can ease integration of future policy changes and gain an edge over competitors slow to adopt more ethical practices,” says Almansoori. “Building the foundation is key.”
Questions to ask:
|
Perspective
Considered the first comprehensive regulations governing AI, the EU AI Act will significantly impact how CSPs update their governance. The Act requires organizations to ensure AI applications that are deemed “high risk” are safe, transparent, and adhere strictly to privacy laws. But beyond the EU AI Act, CSPs must manage a complex web of AI regulations that are also being established across jurisdictions—from countries down to even the state level in the US. For example, Canada is advancing an AI and Data Act, India has proposed the Digital India Act, China is introducing multiple governance initiatives, while Australia is emphasizing current regulatory structures for AI oversight.
e&’s Almansoori cites several examples of how regulations will apply to CSPs: demonstrating how chatbots arrive at their responses, avoiding the generation of deceptive or misleading marketing messages or exploitation of user data, and being able to explain how an AI system predicts network traffic patterns and allocates resources.
CSPs will need to establish legal teams to track the evolution and impact of AI regulations. Tech companies are also building governance and automation into gen AI solutions to help ease the burden.
![]() | 6. Assess and address skills needs. |
Respondents cite inadequate expertise as one of the top barriers to generative AI adoption, but from e&’s Almansoori’s perspective, the issue is deeper. “Strategic talent acquisition and upskilling is a challenge, and those getting ahead are proactively cultivating internal centers of AI excellence rather than relying on hiring alone,” she notes. “They are investing in reskilling talent.”
e& models this approach with their AI Competency Hub and Citizen X program, where employees receive extensive training opportunities. These include courses in AI fundamentals plus hands-on experience developing solutions or innovating with AI products. e& also launched an AI Graduate Program in 2021 to help ensure all new-graduate hires are skilled in AI fundamentals regardless of job placement or background. Similarly, NTT’s Komazawa says that to encourage interest, they have started an AI Working Committee where use cases, governance, and security of gen AI can be discussed.
As with previous technology (such as cloud or network infrastructure), CSPs can rely on business partners to help bridge the AI skills gap. In fact, our respondents are banking on that: 74% say current ecosystem partners are essential for their gen AI innovation efforts. 76% are also re-valuating ecosystem partnerships to get more innovation value from generative AI.
Technology partners can offer specialized AI solutions that don’t require CSPs to overhaul their legacy infrastructure. However, Almansoori cautions against overreliance on partners. “Excessive dependency on specific vendors' proprietary technologies and solutions can limit long-term strategic flexibility and control within dynamic markets,” she explains. “Additionally, without a coordinated effort to build AI capabilities internally, telcos may struggle to achieve the scale needed.”
A director at an Asian mobile operator notes the importance of building internal expertise. “In-house technical capabilities are essential,” he says. “Even if we ask our business partners to do the practical work, we must have the technical skills to understand and manage the tasks done by the business partners.”
Telecom leaders also recognize that employees’ apprehension about AI can challenge implementation. “Organizational inertia, lack of prioritization, and resistance to change can significantly impede AI adoption,” Almansoori says. “This includes not just the technological shift but also changing the company culture to embrace data-driven decision-making, redefining job roles, and addressing employee expectations and fears about job displacement.”
Almansoori notes that e&’s goal is to “ensure a responsible transition that leverages gen AI to create new opportunities in addition to reshaping existing roles. Rather than viewing it as a dichotomy between humans and AI, it's more accurate to see it as complementary forces that augment each other's capabilities.”
Questions to ask:
|
![]() | 7. Define a plan for monitoring and adapting. |
Finally, how will a CSP know if its gen AI solution is delivering results as expected? This point on the roadmap can’t be lost in the rush to adoption.
They can start by revisiting the key metrics defined in the business case and checking performance against them continuously. Leaders should take the time to conduct a retrospective review of what went well, what didn’t, and what changes are needed.
Most importantly, given how quickly generative AI is evolving, CSPs must plan to stay up to date on the latest advancements and how those affect current and future use cases. NTT’s Komazawa says this is critical to successful gen AI adoption. “One should carefully monitor the services that occur in the world of generative AI,” he says. “Monitor the market carefully and find out whether new services are provided safely or not.”
At the same time, he encourages a mindset that’s willing to accept a challenge. “Without being open to the challenges of new technologies, it will be difficult to provide innovative services,” he says.
Questions to ask:
|
1 Based on IBM internal benchmarks.
Meet the authors
Rahul Kumar, Senior Partner and Vice President, IBM Consulting Global Industry Leader for Telecom and MediaEoin Coughlan, Global CTO, Telecom, Media, and Entertainment industry, IBM
Priya Kurien, Global Telecommunications, Media, & Entertainment Leader, IBM Institute for Business Value
Originally published 29 May 2024